Wikipedia:Хэрэглэгчид
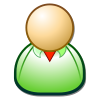
Хэрэглэгчид - гэдэг нь техник хангамжийн бүрэн бүтэн байдлыг хариуцдаг Википедчин юм. Нь хэрэглэгч Википедиагаас.
Энэ өгүүлэл дутуу дулимаг бичигджээ. Нэмж гүйцээж өгөхийг хүсье.
Хэрэглэгчид - гэдэг нь техник хангамжийн бүрэн бүтэн байдлыг хариуцдаг Википедчин юм. Нь хэрэглэгч Википедиагаас.
Энэ өгүүлэл дутуу дулимаг бичигджээ. Нэмж гүйцээж өгөхийг хүсье.